Former NASA engineer and YouTube powerhouse Mark Rober has ignited fresh controversy in the autonomous vehicle world with his latest viral experiment. The video, titled “Can You Fool a Self-Driving Car?”, pits Tesla’s camera-based Autopilot system against Luminar’s LiDAR technology through a series of increasingly challenging scenarios.
With over 15.6 million views and counting, Mark’s test has reopened the long-standing debate about which sensor technology will ultimately prove superior for autonomous driving. Video has drawn responses from industry engineers, AI experts, and Tesla enthusiasts worldwide who question both the methodology and real-world applicability of the tests.
Mark’s experiment consisted of six different test scenarios designed to challenge the perception systems of both vehicles:
- A child crash test dummy on a sunny day
- A partially obscured “peek” dummy
- A child dummy under simulated heavy fog and rain
- Testing under strong backlighting conditions
- A fake wall designed to deceive vision systems
The final scenarioâthe fake wallâhas become the most contentious part of the entire experiment. Critics argue this represents an artificial edge case that drivers would never encounter in real-world conditions, making it an unfair test of the systems’ capabilities.
An important distinction that many viewers missed is that Mark wasn’t actually testing Tesla’s FSD capability, but rather its more limited Autopilot system. Meanwhile, the Luminar-equipped vehicle had additional advantages, including a Luminar employee in the passenger seat during testing.
This discrepancy has led many to question whether the comparison provides meaningful insights about the relative merits of vision-only versus LiDAR-enhanced autonomous driving systems.
Following the video’s release, a former Google software engineer applied a monocular depth estimation model called DepthAnythingV2 to the fake wall scenario. The results showed that even this deceptive visual cue could be accurately identified using advanced vision algorithms.
Further verification came when users consulted large language models including Grok-3 and ChatGPT-4o, both of which correctly identified the wall as an artificial visual deception rather than a real obstacle.
These findings suggest that the vision vs. LiDAR debate isn’t simply about hardware limitations, but also about the sophistication of the software interpreting sensor data.
Adding fuel to the fire, Tesla FSD senior engineer Yun-Ta Tsai commented that the scenarios presented by Chinese FSD owners in their independent testing are “far more interesting than those showcased by NASA engineers.”
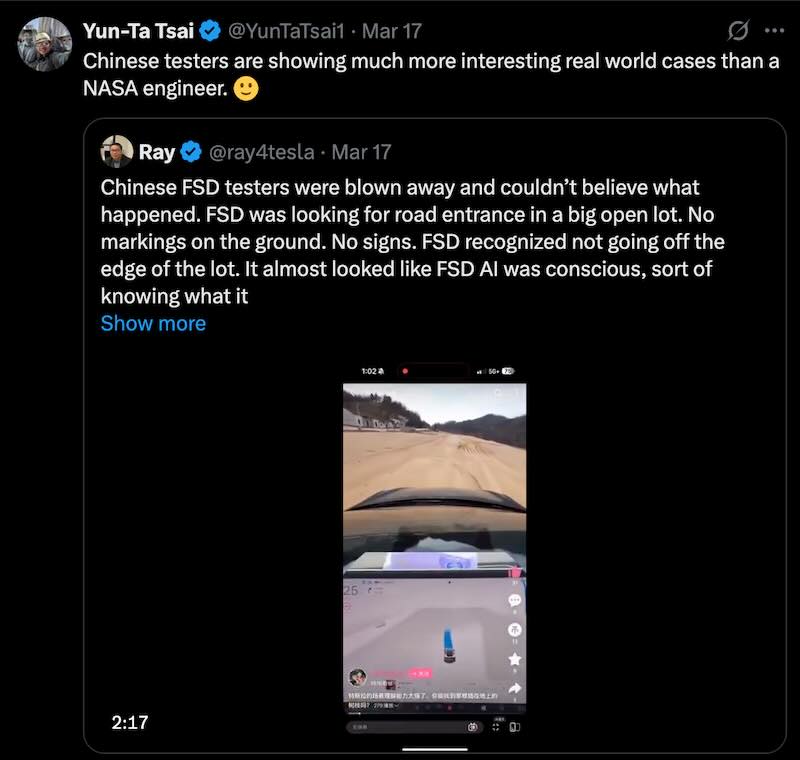
Tesla AI Sr. Staff Engineer: Chinese testers are showing much more interesting real world cases than a NASA engineer.
This remark highlights the global nature of autonomous vehicle development and suggests that real-world testing across diverse environments may be more valuable than controlled experiments like Mark’s.
Chinese Tesla owners have been conducting their own FSD tests in challenging conditions. One video shows FSD successfully navigating around abandoned tires in low-light conditions at speeds ranging from 50 to 100 km/h.
In another community test, a transparent film wall was set up during daylight hoursâa scenario arguably as extreme as Mark’s fake wallâyet Tesla’s FSD system correctly identified and avoided it without issue.
These community tests provide additional data points that suggest vision-based systems may be more capable than Rober’s experiment indicates.
Industry experts increasingly believe that as both technologies mature, the performance gap between pure vision systems and multi-sensor fusion approaches will narrow significantly.
This convergence theory suggests that eventually, there will be no practical difference in how well vision-only and LiDAR-inclusive systems perform across typical driving scenariosâwhether on highways, in construction zones, or navigating complex urban environments with dynamic obstacles.
Beyond technical capabilities, the economic aspect can’t be ignored. Camera-based systems typically cost significantly less than LiDAR setups, which has been a key factor in Tesla’s strategy. If vision systems can achieve parity with LiDAR in terms of safety and reliability, the cost advantage could prove decisive for mass-market adoption.
However, proponents of LiDAR argue that the additional safety margin provided by redundant sensing technologies justifies the higher cost, especially during the transition period when autonomous systems are still evolving.
As the Vision vs. LiDAR debate heats up following Mark’s controversial test, the autonomous vehicle industry continues to develop both approaches in parallel. Regulatory bodies are watching closely, as their decisions about required safety standards could ultimately influence which technologies dominate.
What’s becoming increasingly clear is that software algorithmsânot just hardware sensorsâwill play a crucial role in determining the winner of this technological race. As machine learning models improve, even basic camera systems can achieve remarkable perception capabilities that were previously thought to require specialized sensors.
Whether pure vision can truly handle all perception requirements for driving remains to be seen, the road to fully autonomous vehicles will involve many more tests, debates, and technological leaps before reaching its destination.
Related Post
Tesla FSD v13.2: Vision-Based, A Paradigm Shift in Autonomous Driving Technology
Baidu IDG Chief R&D Architect Wang Liang on Tesla FSD V12 and LiDAR vs Vision
Beyond Lidar: How Tesla Vision-only E2E and MobilEye Radar Are Reshaping Autonomous Driving